National Institute of Technology, Silchar (NITS) Speech and Image Processing Laboratory & The LNMIIT, Jaipur
(Department of Electronics and Communication Engineering, NIT Silchar, Assam, India & The LNMIIT,Jaipur,India)
Project by: Dr. Joyeeta Singha (Asst. Prof. , ECE Department, The LNMIIT), Songhita Misra (PhD Scholar, ECE Department, NIT Silchar), Dr. R.H. Laskar (Asst. Prof. & Head, ECE Department, NIT Silchar), Shweta Saboo (PhD Scholar, ECE Department, The LNMIIT), Manoj Sain (PhD Scholar, ECE Department, The LNMIIT)
1. NITS Hand Gesture Database I (no variation in gesticulation speed or pattern)
Database Description: The database consist of 40 different hand gesture classes which included
-
Ten Numbers- Zero to Nine
-
Twenty Six Alphabets- A to Z (Upper Case)
-
Four Arithmetic Operations- Addition, Subtraction, Divide, Multiplication
The gestures were collected from 20 users and they donot have any prior experience with the mid-air gestures. The users are the M.Tech and Ph.D students of ECE Department, NIT Silchar who are related to Speech, Image and Video processing. They have good knowledge about the Signal Processing and related areas.
The gestures were recorded from 20 different subjects with a webcam having 1MP HD and resolution of [1280 X 720]. A total of 8000 samples were collected: 6000 samples for training (Each gesture with 150 samples) and 2000 samples for testing (Each gesture with 50 samples). Some of the video frames for few gestures are shown below. The datasets were collected in both simple and complex background. Few of the database can be downloaded from the link given here: . For full database contact us using the message box given at the end of the page.
The database has been extended to 10,000 gestures later.
2. NITS Hand Gesture Database II (pattern variation)
It consists of 6000 gestures. Here the users are allowed to gesticulate in natural pattern whereas in Database I, the users were restricted to gesticulte with the fixed pattern they were given with. We have tried to address few of the variations. The variations are shown from the gestures provided in the link given here: . For full database please contact us.
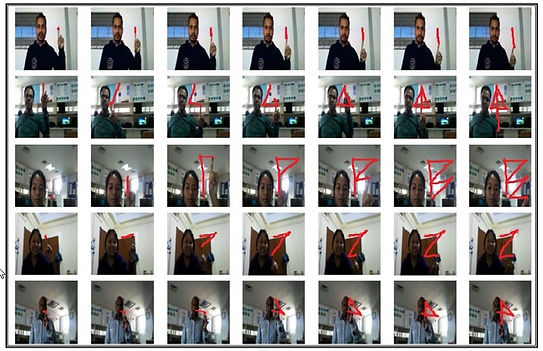
3. NITS Dynamic Hand Gesture Database III (speed variation)
This set of database includes the variation in the speed of the hand of users during gesticulation. Sample of this database is provided in this link.
4. NITS Hand Gesture Database IV (bare hand)
This set of database includes the hand gestures (40 classes as described for Database I) using bare hands. The reference set of gestures are provided in this link.
The users were asked to gesticulate keeping the following conditions:
-
The background should not consist of moving skin colored objects at the start of the video recording.
-
The hand should be already available at the start position of the gesture before the video recording starts.
-
The palm of the hand should be moving at the same place for few seconds to detect the presence of hand.
-
The hand is then moved smoothly and slowly to the most prominent gesture positions.
-
The hand is kept in the final gesture position for few seconds to complete the gesture.
5. NITS Hand Gesture Database V (continuous hand gesture)
This set of database includes the continuous sequence of hand gestures using bare hands. The length of the sequence may varry from 1 to 10.
6. NITS Hand Gesture Database VI
This set of database includes the extra 18 set of gestures compared to our hand gesture database I. This set of gestures include (~, @, =, ^, &, }, {, ], [ , ), (, \, ?, %, <, >, $, !). The reference set of gestures are provided in this link.
7. NITS Hand Gesture Database VII
This set of database includes the bare hand dataset. The set of gestures used is same as Database VI. The samples of this database is provided in the link.
8. LNMIIT Dynamic Hand Gesture Dataset-1
This set of database includes the dataset with challenging conditions like Change in hand shape, Illumination variations and presence of multiple persons in indoor environment. The samples of this database is provided in the link.
9. LNMIIT Dynamic Hand Gesture Dataset-2
This set of database includes the gestures of numerals and lower case alphabets. The samples of this database is provided in the link.
10. LNMIIT Dynamic Hand Gesture Dataset-3
This set of database includes the gestures of Numerals, Lower Case and Upper Case Alphabets with Self-co-articulation strokes. The samples of this database is provided in the link.
11. LNMIIT Dynamic Hand Gesture Dataset-4
This set of database includes the gestures of Numerals, Lower Case and Upper Case Alphabets with Self-co-articulation strokes and pattern variation. The samples of this database is provided in the link.
12. LNMIIT Dynamic Hand Gesture Dataset-5
This dataset consists of different gestures including numerals and alphabets recorded in dynamic environments like peresence of extra person and hand, indoor and outdoor environments. Few samples are provided in the link below.
13. LNMIIT Dynamic Hand Gesture Dataset-6
This dataset includes some of the word level gestures like "12", "ON" etc. The gestures include self co-articulation, pattern variation and movement epenthesis. Some of the samples are provided in the link given below.
14. LNMIIT-KHAD--RGB
15 LNMIIT-KHAD Skeleton Dataset
This dataset includes RGB video sample for Human activities such as Eating, Handshake, Headache, Exercise, vomiting, sit-ups, walking.
This dataset includes skeleton video sample for Human activities such as Eating, Handshake, Headache, Exercise, vomiting, sit-ups, walking.
If you are using this NITS dynamic hand gesture database, please cite these papers:
1. J. Singha, R.H. Laskar, “Self co-articulation detection and trajectory guided recognition for dynamic hand gestures”, IET Computer Vision, vol. 10, no. 2, pp. 143-152, 2015, DOI: 10.1049/iet-cvi.2014.0432.
2. J. Singha, R.H. Laskar, “ANN-based hand gesture recognition using self co-articulated set of features”, IETE Journal of Research, vol. 61, no. 6, pp. 597-608, July 2015, DOI: 10.1080/03772063.2015.1054900 (Taylor and Francis)
3. J.Singha, R.H. Laskar, “Recognition of global hand gestures using self co-articulation information and classifier fusion”, Journal on Multimodal User Interfaces, vol. 10, no. 1, pp. 77-93, January 2016, DOI: 10.1007/s12193-016-0212-0. (Springer)
4. J. Singha, S. Misra, R.H. Laskar, “Effect of variation in gesticulation pattern in dynamic hand gesture recognition system”, Neurocomputing, vol. 208, 2016, pp. 269-280, DOI: 10.1016/j.neucom.2016.05.049. (Elsevier)
5. J. Singha, A. Roy, R.H. Laskar, "Dynamic hand gesture recognition using vision-based approach for human–computer interaction", Neural Computing and Applications, August 2016, pp. 1-13, DOI: 10.1007/s00521-016-2525-z (Springer)
Modified KLT results of few video sequences of IBGHT database
We performed an experimentation on some of the video sequences of IBGHT database [14] such as ‘indoor_1’, ‘indoor_3’, ‘indoor_4’, ‘outdoor_4’, ‘scene_C’, ‘scene_D’ and ‘scene_E’ . The comparative results of our algorithm with existing techniques has been provided for both the stages (hand segmentation and hand tracking).
a) Comparative results for hand segmentation (Fig. 1):
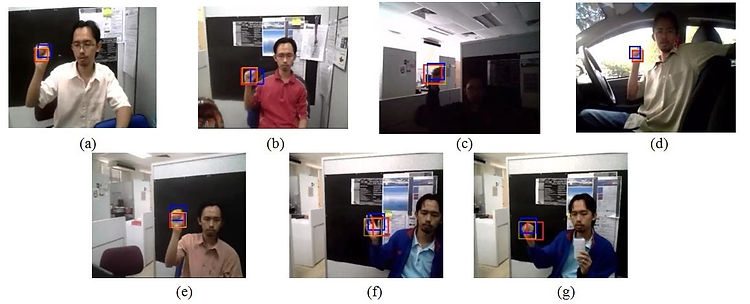
Fig. 1 Hand detection for video sequence (Top) (a) ‘indoor_1’ (b) ‘indoor_3’ (c) ‘indoor_4’ (d) ‘outdoor_3’. (Bottom) (e) ‘scene_C’ (f) ‘scene_D’ (g) ‘scene_E’ (red: [15], blue: [9], yellow: proposed)
b) Comparative results for hand tracking (Fig. 2):

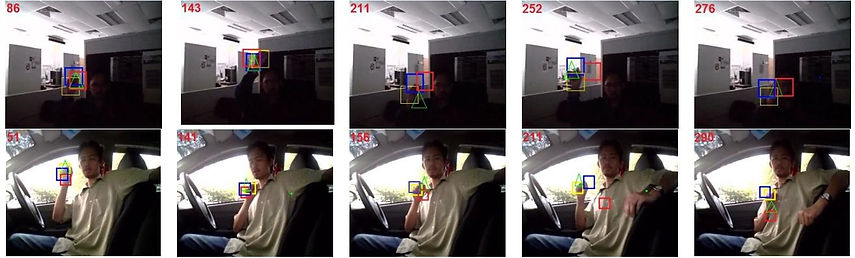


Fig. 2 Top to bottom: indoor_1, indoor_3, indoor_4, outdoor_3, scene_C, scene_D, and scene_E video sequences. Left to right: Results of Camshift [16], KLT [10], AKFIE [9] and proposed tracking system for different video sequence (red: [16], blue: [10], green: [9], yellow: proposed)
References:
[9] Asaari, M.S.M., Rosdi, B.A., Suandi, S.A.: ‘Adaptive Kalman Filter Incorporated Eigenhand (AKFIE) for real-time hand tracking system’, Multimed. Tools Appl., June 2014, 70, (3), pp. 1869-1898.
[10] Kolsch, M., Turk, M.: ‘Fast 2D hand tracking with flocks of features and multi-cue integration’, in Proc. IEEE Conference on Computer Vision and Pattern Recognition Workshop, June 2004, pp. 158.
[14] Asaari, M.S.M., Rosdi, B.A., Suandi, S.A.: ‘Intelligent Biometric Group Hand Tracking (IBGHT) database for visual hand tracking research and development’, Multimed. Tools Appl., 2012, 70, (3), pp. 1869-1898.
[15] Choudhury, A., Talukdar, A.K., Sarma, K.K.: ‘A Conditional random field based Indian Sign Language recognition system under complex background’, in Proc. Fourth IEEE International Conference on Communication Systems and Network Technologies, April 2014, pp. 900-904, Bhopal.
[16] Nadgeri, S.M., Sawarkar, S.D., Gawande, A.D.: ‘Hand gesture recognition using Camshift algorithm’, in Proc Third IEEE International conference on Emerging Trends in Engineering and Technology, 2010, pp. 37-41, Goa.